At any particular point in time, the state of the world – such as energy use, carbon emissions, gender-disaggregated educational attainment and labour force participation, or the movement of people – can be represented in an artificial intelligence (AI) system called a knowledge graph. Knowledge graphs are networks of data (concepts and objects) expressed as a graph. If a knowledge graph (KG) is sufficiently comprehensive, it can be employed to test and trial nearly unlimited scenarios for better real-world decision making.
This kind of representative knowledge graph employs “symbolic” AI (sometimes referred to as “good old-fashioned AI” – GOFAI). One advantage of this method is that unlabelled data is given meaning by semantic tagging. This allows agents, software programs that respond on behalf of the knowledge graph’s owner, to operate on the knowledge graph. However, as this process relies on human labour, it can be time-consuming and cumbersome.
One way to mitigate this is to integrate human-guided machine learning algorithms into AI so that the knowledge graph can learn as it develops. CARES’ World Avatar Project, a dynamic knowledge graph, exemplifies this approach and allows us to imagine new worlds and scenarios. The World Avatar Project was started in 2013 and aims to create a digital avatar of the real world that connects data and computational agents. These agents incorporate real-time data, which is analysed to optimise decision-making across complex systems. We currently make use of data across different domains – cities, infrastructure, industry, production, transport and more – to test various scenarios and identify best solutions for greater energy efficiency and carbon reduction.
Image by Gerd Altmann from Pixabay
Although machine learning algorithms bring greater functionality and predictive power to a knowledge graph, they are trained with data and can therefore be problematic as they represent the world in a state that we may not want to emulate, for example, perpetuating racial and gender biases when predicting the probability of people getting hired. Furthermore, these algorithms do not have a particular goal; they simply act on the data they are given. Someone must decide which direction a machine learning algorithm should take, so a knowledge graph representing an AI needs to contain language that represents goals as well as data.
CARES World Avatar project deliberately integrates human goals and concepts into its AI system to achieve this. We combine the best parts of symbolic AI with modern machine learning to create an ever-evolving, dynamic system with better outcomes.
How should we decide which goals to integrate into our AI systems? While most people agree that carbon emissions need to be reduced, there is a huge variation of proposed solutions and methods to achieve this. As we have learned the hard way from the COVID-19 pandemic, a drastic reduction in greenhouse emissions is certainly possible (due to the decline in human and economic activities as a result of near-global lockdowns), but this cost us in many other dimensions including health, jobs and food security, with disproportionate impacts on women. Which actions should be prioritised and how quickly can all of this happen?
There are many different paths to take here, but CARES has chosen to start with the UN Sustainable Development Goals (SDGs). Although ethics and human concepts are too complex for any set of systems to capture perfectly, the SDGs provide a blueprint for a more equal, sustainable and inclusive future, and are globally agreed upon. The overarching focus of most CARES research programmes is the reduction of carbon emissions to help Singapore – and the world – meet its Paris Agreement pledge. This links in with several of the SDGs, in particular Goal 7 (affordable and clean energy) and Goal 13 (climate action). The UN has noted that climate change threatens to undermine many of the development gains made over the past few decades, making it a particularly urgent issue to solve.
The United Nations Sustainable Development Goals
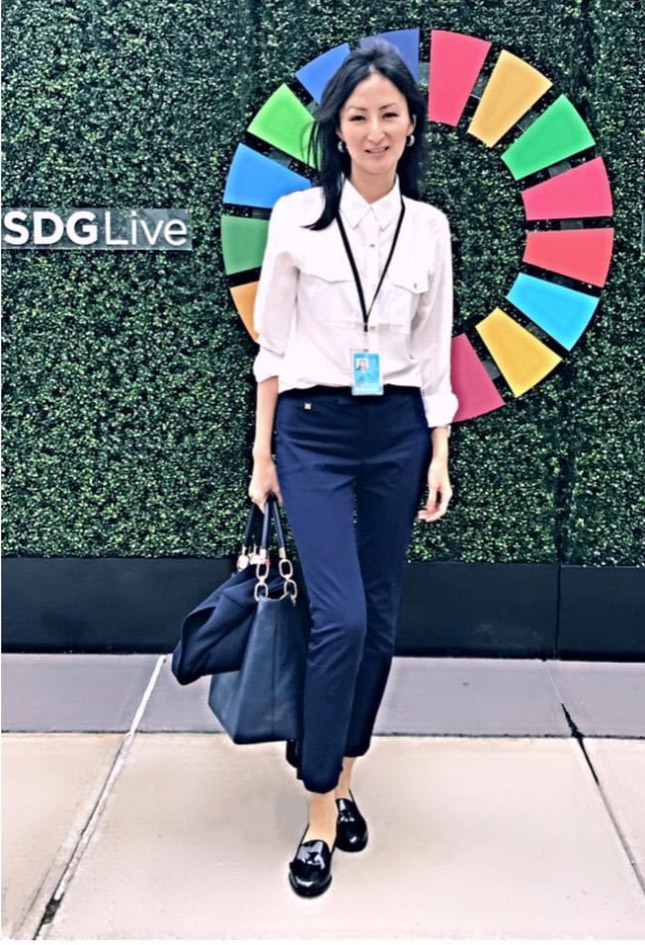
Dr Esuna Dugarova. Photo: Supplied
As part of this effort, CARES is proud to engage with Dr Esuna Dugarova, a Cambridge graduate (PhD, Churchill College) and a policy specialist at the United Nations Development Programme.[1] Originally from Buryatia, Dr Dugarova leads analysis and research on sustainable development with a focus on gender equality, social protection and poverty reduction. She is a research coordinator of the COVID-19 Global Gender Response Tracker which has analysed over 2,500 policy measures in response to COVID-19 in 206 countries and territories. It has found that the global policy response to the pandemic has largely been blind to gender equality, with 70% of gender-sensitive measures addressing violence against women and only 18% targeting women’s economic security and unpaid care work. The tracker is being updated with new policy measures, including on green recovery.
With Dr Dugarova’s support, two students at the University of Cambridge are looking into how the SDGs can be represented in a knowledge graph so that its agents can operate in line with the global goals. This is an exciting first step to explore the integration of human concepts and development goals with AI systems so that we can build a better virtual, and real, world.
[1] This engagement is done in her personal capacity, and the views presented during this process are hers and do not necessarily reflect the views of the UN system.